Making artificial intelligence count for science – CSIRO
AUSTRALIA’S NATIONAL SCIENCE AGENCY We’re using artificial intelligence (AI) responsibly in our science to help us count tiny things
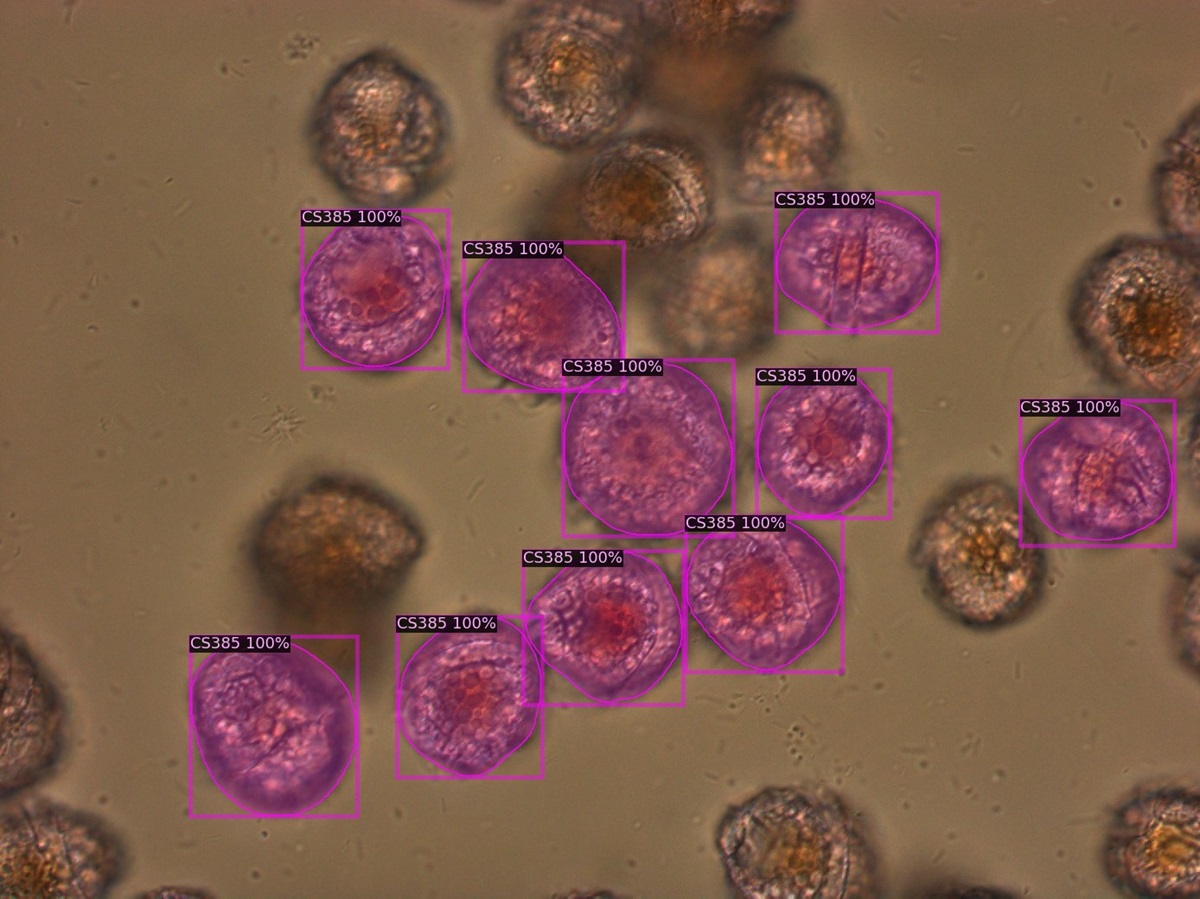
AUSTRALIA’S NATIONAL SCIENCE AGENCY
We’re using artificial intelligence (AI) responsibly in our science to help us count tiny things with big impact.
Share
There’s been a lot of talk about how AI will transform the way we work.
Whether you see AI in the workforce as a friend or foe, there is no doubt this technology will change some jobs as we know them. This includes jobs in the world of science. Our researchers are exploring and responsibly embracing the use of AI in our work.
AI is a particularly useful tool to overcome pesky biological limitations. For example, the human eye can’t distinguish objects smaller than about 0.2mm. Traditionally, we use microscopes to overcome this. But once an object or substance is magnified, a highly-trained expert still needs to look at it to identify and count items of interest. Counting specimens or objects for science can be a slow, labour-intensive, and costly process.
Here are a couple of examples where we’ve leveraged the impressive advances in machine learning over the past decade to make AI count. These projects were advanced by our Machine Learning and Artificial Intelligence Future Science Platform, which unites collaborators from across the organisation to advance machine learning for scientific discovery.
Working with our experts in agriculture, we’ve created a model that can quantify the number of hairs on the back of a cotton leaf.
Leaf hairiness impacts the insect resistance, fibre yield and value of new varieties of cotton. Traditionally, it’s eyeballed by experts in commercial breeding programs and given a score between one and five.
First we developed AI models which can generate the same hairiness scores as humans with 95 per cent accuracy.
Dr Moshiur Farazi is one of our experts in computer vision, focusing on enabling computers to identify and understand objects in images and video. He said HairNet2 is now moving beyond automating current methods.
“Training models to reproduce human ratings of hairiness can increase the speed and scale of analysis. However, these models reproduce the variability in the human estimates they’ve been trained on,” Moshiur said.
“In HairNet2 we’ve created a new method of AI-augmented scoring which is more robust, reliable and accurate.
“This model estimates the area of the leaf that is covered by hairs by locating all hairs on the leaf, something that is not completely impossible for a human to do, but incredibly difficult and time consuming.
“HairNet2 was trained using about 1000 images where humans had annotated every single hair. This laborious annotation process helped build an AI tool that can automate the hairiness scoring beyond human perception,” he said.
The new models are being deployed on a web interface for breeders to test during the next cotton season. You can try an early demo yourself.
Harmful algal blooms are large populations of algae that can be toxic for both humans and animals.
To identify harmful algal blooms, experts undertake extensive testing using a microscope and a counting chamber (a slide with precise gridlines allowing scientists to estimate the number of harmful algal cells in a liquid sample).
Dr Chris Jackett is an expert in object detection. He began working to augment this manual process with AI as a postdoctoral research fellow with our National Research Collections Australia.
“It’s an enormously time-consuming and labour-intensive exercise, and humans are limited in the number of samples they can process,” Chris said.
“Extended sessions at the microscope can also lead to health issues like vision problems, bad posture, physical strain, and headaches.”
In response to this challenge, we are training machine learning models to automatically detect harmful algae in images.
Our team is systematically photographing and annotating algae strain samples from the Australian National Algae Culture Collection. We are also beginning to use a range of AI tools to help speed up the annotation process.
With this combined human/computer effort, we have built up an annotated dataset for 15 different strains of algae to-date, which are now being used to train the AI models. Early testing suggests these models can successfully detect the target strains with a high level of accuracy.
Using AI to provide faster and more accurate detection of toxic algae could have significant economic, environmental and social impacts.
“Improving the speed and accuracy of harmful algae detection could provide an early warning signal for water managers indicating when and where blooms might occur,” Chris said.
AI-enhanced risk management and decision-making could help safeguard the health of the environment as well as coastal communities, consumers and Australian fisheries and aquaculture businesses.
Many organisations are currently grappling with the potential of AI to transform their processes and business.
If your core business involves counting or identifying objects, AI may be a friend.
Moshiur said the barrier to entry for those looking to implement AI for object detection is low.
“Five or 10 years back you needed to train the model on your own and needed a lot more data and compute power to experiment with AI,” Moshiur said.
“If you have a very small amount of data, you can fine-tune open-source models to work on your problem with as little as a couple of hundred images.”
However, he said the successful application of AI depends on asking the right questions.
“At the end of the day, most users want a black box where they can click buttons and get the desired answer. But we need to unpack what they want the buttons to do and get the data ready in a way that enables those buttons to arrive at the right answer,” Moshiur said.
“Taking time to sit down and explore the questions you want answered, and consider the problems you haven’t been able to solve with human-driven methods or processes, is the best place to start.”
We are committed to child safety and to the implementation of Child Safe principles and procedures.
Thanks. You’re all set to get our newsletter
We could not sign you up to receive our newsletter. Please try again later or contact us if this persists.
CSIRO acknowledges the Traditional Owners of the land, sea and waters, of the area that we live and work on across Australia. We acknowledge their continuing connection to their culture and pay our respects to their Elders past and present. View our vision towards reconciliation.
Find out how we can help you and your business. Get in touch using the form below and our experts will get in contact soon!
CSIRO will handle your personal information in accordance with the Privacy Act 1988 (Cth) and our Privacy Policy.
Enter a valid email address, for example jane.doe@csiro.au
A Country value must be provided
First name must be filled in
Surname must be filled in
Please choose an option
Organisation must be filled in
Please provide a subject for the enquriy
0 / 100
We'll need to know what you want to contact us about so we can give you an answer
0 / 1900
We have received your enquiry and will reply soon.
The contact form is currently unavailable. Please try again later. If this problem persists, please call us with your enquiry on 1300 363 400 or +61 3 9545 2176. We are available from 9.00 am to 4.00 pm AEST Monday – Friday.